Tech
Generalizing Rao’s Contributions to Information Geometry in Future Quantum Computing Contexts: A (Very) Preliminary Analysis for Technology Experts.

Published
6 years agoon
By
Imran Javed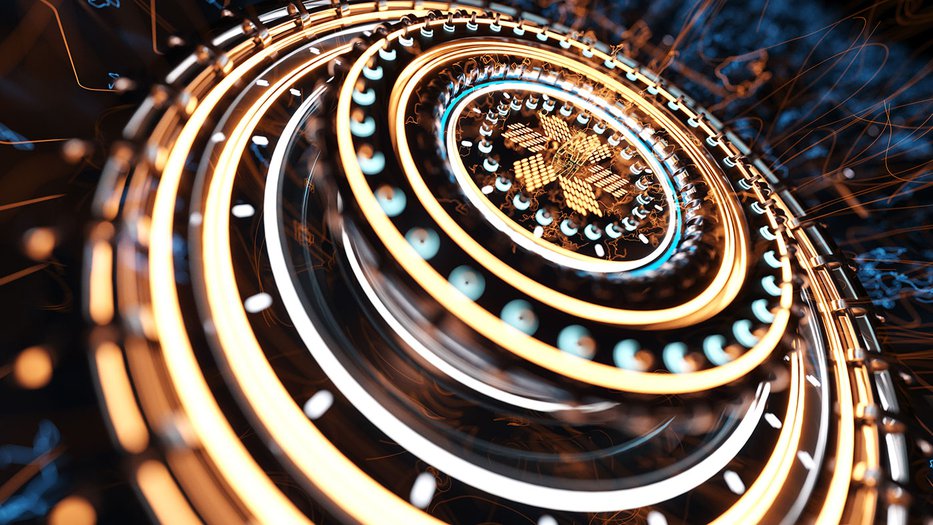
Dr. Jonathan Kenigson, FRSA
- R. Rao laid the foundation for modern Information Geometry in 1945 while a research student at Andhra and Calcutta – a task that involved the employment of Manifold Theory in conditions of divergence and entropy. The initial ideas employed in the paradigm involve: (1). Positive-Definite Information Matrices as Norms on Function Spaces; (2). Function Spaces that are restricted to the context(s) of spaces of manifolds of Distribution Functions. In particular, (2) assumes the form of spaces of Normal Distributions, whose global geometry is isomorphic to the “Upper-Half-Plane” Model of Hyperbolic Geometry with the classical Poincaré metric. In a “qualitative” sense, the distinction between two distributions may be interpreted as a proportion of the difference of their relative Information Entropies (IE). This interpretation lends itself well to modern computation and AI because such entropies, while long-known, have just begun to be explored in Quantum contexts. Information from network topologies and architectures explored from categorical contexts are devoid of this expression. In the former paradigm, entropy cannot be quantified in an integrable sense because of the lack of a measure-theoretic expression of the relevant categorical quantities.
The Riemannian structure of the Information Manifold, however, renders it a Topological Space, and, more particularly, a “Metric Space,” whose distance function is positive-definite and derived from the concavity conditions implied in the “Fisher-Rao Information Matrix.” Any Information Manifold also possesses a “coherent” integral structure whose “Lebesgue-Measurable” sets are determined canonically by the “Ring of Outer Measures” on the manifold. The resulting Lebesgue Integral has properties that permit efficient computation as guaranteed by abstract Measure Theory – namely, arbitrary pointwise approximation by binary step functions and strong restrictions on information convergence demanded by such results as Fatou’s Lemma and the Lebesgue Dominated Convergence Theorem.
Entropies that generalize the Rényi entropy (like the Kullback-Leibler Divergence), may be constructed on the tangent spaces of an Information Manifold as Surface Integrals. The resulting paradigm permits novel applications of Ergodic metrization. Categorical and Measure-Theoretic notions may be introduced induced through integral Prokhorov metrization and then rendered algebraically by the introduction of Markov Transition Kernels between the manifolds. This approach retains the rich geometry of Rao’s paradigm without sacrificing the expediency and applicability of Categorical notions of transition functions. Given a countable sequence of random variables on a probability space (that are not necessarily identically distributed), one can employ Fisher-Rao theory to construct a sequence of Information Manifolds in the metric tensor defined by the Fisher Information. This manifold sequence is associated with a sequence of spaces of probability measures defined on the Borel subsets induced by each metric. Each of these is capable of Prokhorov Metrization. We introduce a modern measure theory to rigorously define transition kernels between the Fisher-Rao Manifolds, associating a generalized Markov process with each transition.
I present herein a paradigm for this attempt that could permit generalization of existing results to the study of Information Manifolds generated in Quantum Computing – in which, among other differences, the underlying manifolds are not comprised of Normal Distributions but of Wavefunctions from some underlying computational process that generates functional data capable of at least positive-semidefinite metrization in the manner of a Riemannian Manifold. Mathematically, the generalized Kolmogorov Central Limit Theorems are sufficiently robust to permit strong statements about Convergence in Law that generalize the classical CLT to situations in which the underlying random variables are not Independent and Identically Distributed (IID). Relevant resources for each step of the process are presented below. Initially, one begins with the notion of probability as a derivation on the Lebesgue Measures. Even though it is quite old, the Kolmogorov framework is still extremely useful.
The best reference for this work available in modern translation is likely still Andrei Nikolajevich Kolmogorov’s 1950 masterwork, Foundations of the Theory of Probability (In Russian). The properties of a random variable on a measurable space are lucidly explored by Bert Fristedt and Lawrence Gray’s 1996 text, A modern approach to probability theory published by Birkhäuser in Boston. Convergence results should encapsulate the measure-theoretic properties of the base space in Lebesgue terms so that a general Smooth Orientable Manifold can be created from local spaces. It is the opinion of the current author that the most lucid account of convergence in such generality is furnished in Billingsley, Patrick (1999). Convergence of probability measures. 2nd ed. John Wiley & Sons. pp.1-28. Once an Information Manifold with a given metric tensor exists, one may set about to explore various divergences induced by: (1). Fisher Information and (2). Quasi-Canonical divergences that exist on the tangent spaces, like the Kullback-Leibler Divergence. It is the current author’s opinion that the best resources devoted to this approach are Nagaoka Hiroshi’s 2000 monograph, Methods of information geometry, Translations of mathematical monographs; v. 191, and Shun’ichi Amari’s 1985 introductory applied text Differential-geometrical methods in statistics published by Springer-Verlag in Berlin. Metrization of the spaces of Borel measures on a sequence of Information Manifolds is possible using so-called Prokhorov Processes. Billingsley’s (1999) treatment is particularly salient because convergence on Riemannian Manifolds also induces convergence on sequences of measures, which are themselves defined on the underlying rings of Sigma Algebras for each manifold’s integral structure.
More abstractly, the current author finds that the Fisher Information can be defined on the tangent space S of arbitrary Radon measures using the Radon-Nikodym Theorem. The clearest and most concise existing resource for this treatment may be found in Mitsuhiro Ito and Yuichi Shishido’s 2008 article, “Fisher information metric and Poisson kernels” in the journal Differential Geometry and Its Applications. 26 (4): 347 – 356. The notion of “Poisson Kernel” is intended in the singular sense of a classical Normal Distribution rather than the sense of Kernel-Superpositions that arise in (for instance) Jacobi Theta representations of pure imaginary arguments. From this vantage, one defines an arbitrary f-Divergence on each Statistical Manifold S(X) in the manner of Coeurjolly, J-F. & Drouilhet, R. (2006). “Normalized information-based divergences”.arXiv:math/0604246. A Categorical view of the Markov Kernels and their transition states may be derived intuitively or read from F. W. Lawvere (1962). “The Category of Probabilistic Mappings” by any available publisher. This resource is free in the public domain in the USA. Further submissions will further elucidate the details of the proposed paradigm.
I am a professional blogger/writer and have been writing as a freelance writer for various websites. Now I have joined one of the most recognized platforms in the world.
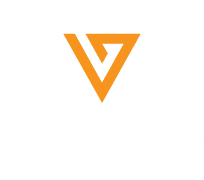
You may like

Achieving Complete Computer Protection: Key Tips for Home Users

Common Questions About Plastic Surgery Prices in the U.S.: Trends, State Comparisons, and Important Considerations

Is Fmovies Safe? What You Need to Know Before Streaming

What You Need to Know About Police Brutality?

12 Sites to Watch Free Online TV Shows with Complete Episodes in 2024
